Heart failure is a growing epidemic in Canada. It is a significant health issue for hundreds of thousands of Canadians and their families, and its reach is expanding. It is often the last stop for Canadians who experience a journey through cardiovascular disease. As Dr. Paul Fedak, a cardiac surgeon at the University of Calgary, explains, “Heart failure is the end result of all cardiac disease. You get heart failure from everything that goes wrong with your heart — all roads lead to heart failure.”
“There is a huge economic cost associated with heart failure,” says Dr. Justin Ezekowitz, director of the Heart Function Clinic at the University of Alberta. “The biggest driver of costs is hospitalisation and emergency room visits.”
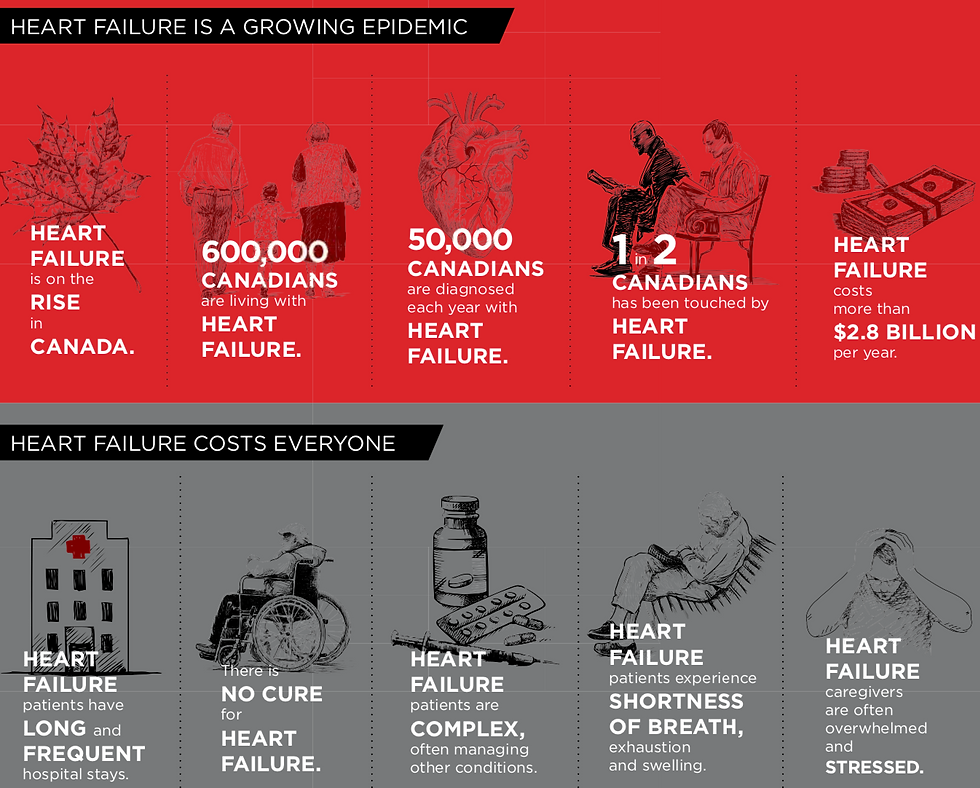
Dr. Fedak points out that diagnosing heart failure is still challenging, especially in the early stages. The longer it takes to diagnose, the more damage is done and the sicker patients eventually become. There are a wide variety of causes which leads to confusion during diagnosis. For instance, Diabetes and high blood pressure are also risk factors, and by controlling these two conditions heart failure can be prevented. Early screening for patients with diabetes or high blood pressure would help diagnose more patients in early stage heart failure.
We were tasked to find if the chances of developing a cardiovascular disease are higher in Canadian adults suffering from osteoarthritis.
Data Source: Canadian Community Health Survey (CCHS) cycles 1.1, 2.1 and 3.1. https://www.dropbox.com/sh/dntqkl6wv54ypop/AACPOf6pnGh4sgithHJRQyYYa?dl=1
Data Description: This survey gathers health-related data for the Canadian population 12 years of age and over, living in the 10 provinces and 3 territories, covering about 97% of the target population. In this study, we have used Public Use Microdata Files from cycles 1.1, 2.1 and 3.1 that contain de-identified data collected in years 2000-2001, 2003 and 2005, respectively. The dataset consisted a total of around 400,000 patients’ responses to about 600-1200 survey questions.
Distribution of dependant variable against the exposure variable:
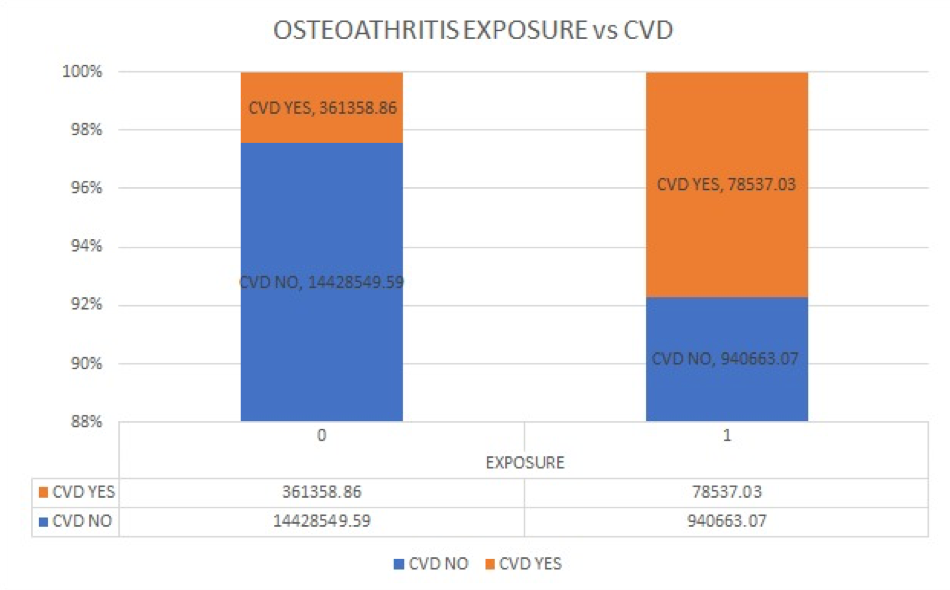
Feature Selection: For the purpose of this case study, we were required to assume that, from the literature, the following variables are risk factors for the outcome and confounders in the relationship between Cardiovascular diseases and Osteoarthritis: age, sex, ethnicity, education, household income, body mass index (BMI), access to a regular medical doctor, smoking habit, alcohol drinking habit, high-blood pressure, and diabetes.
We extracted the features that were common in a list of literature-derived factors linked with the relationship between CVD and osteoarthritis and a list of quantitatively significant features (generated using lasso in R).
Data Modelling: We created multiple models in R for the refined dataset using various techniques such as regression, logistic regression, survey logistic regression, neural networks, random forest, and XG boost.
The two of our best models are described below:
Survey Logistic Regression: We used the Survey package in R.
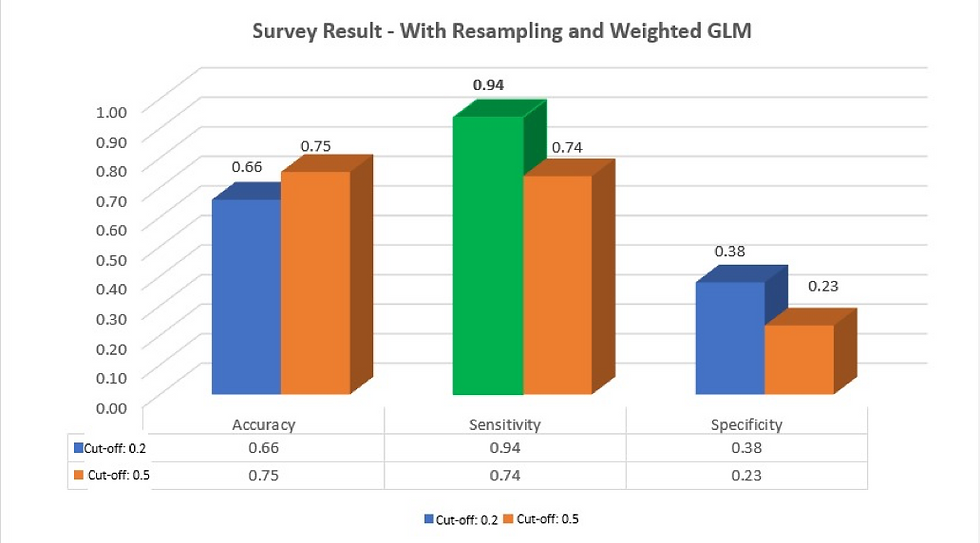
Survey design modeled first.
Change the weights into a weight vector.
Using the function svyglm().
Validation on a test data set using two different cutoffs: 0.2 and 0.5.
XG Boost: We used the XGBoost package in R.
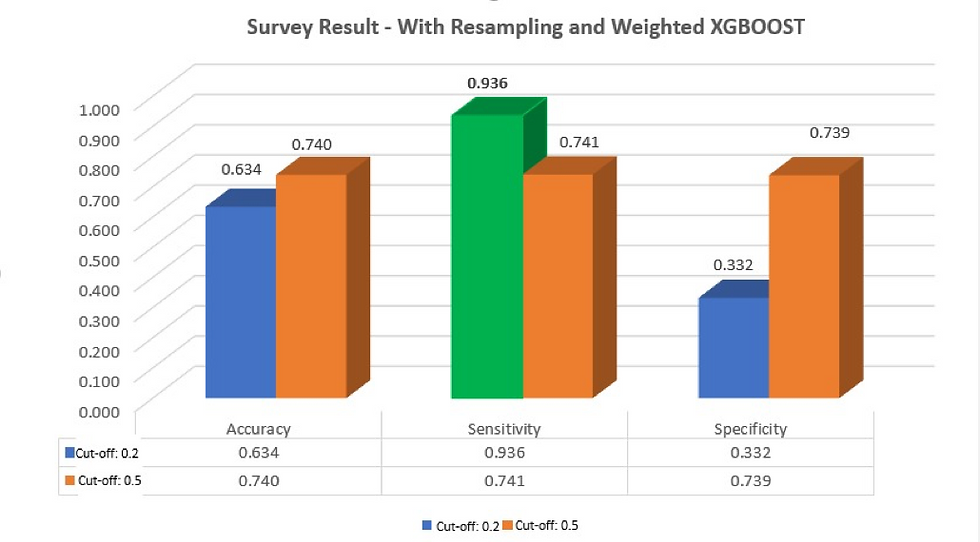
Splitting the training and test data into a label and data.
Data is the transformed into a dense matrix.
A vector of given weights is used while running the model.
Validation on a test data using two cutoffs: 0.2 and 0.5.
Best result at cutoff = 0.5. High sensitivity and good accuracy.
In conclusion we can say with a 74.1% sensitivity that based on the survey data, osteoarthritis patients between the ages 50-64 who have an annual income of less than $15,000 had a three times more chance of getting a CVD than other patients in the same group.
Hence, a pre-emptive screening would have a significant impact on public health and healthcare costs, especially since their healthcare program is centrally funded.
Limitations:
Limited computational capability.
No use of distributed architecture.
Non availability of bootstrap weights and other survey design information, to account for variability correctly.
Split data analysis based on regions, immigration status and other features.
Highly imbalanced response variable.
The Social Challenge
A communication strategy for the research on the relationship between Cardiovascular diseases and Osteoarthritis:

Marketing measures to decrease risks of Cardiovascular diseases among Osteoarthritis patients:
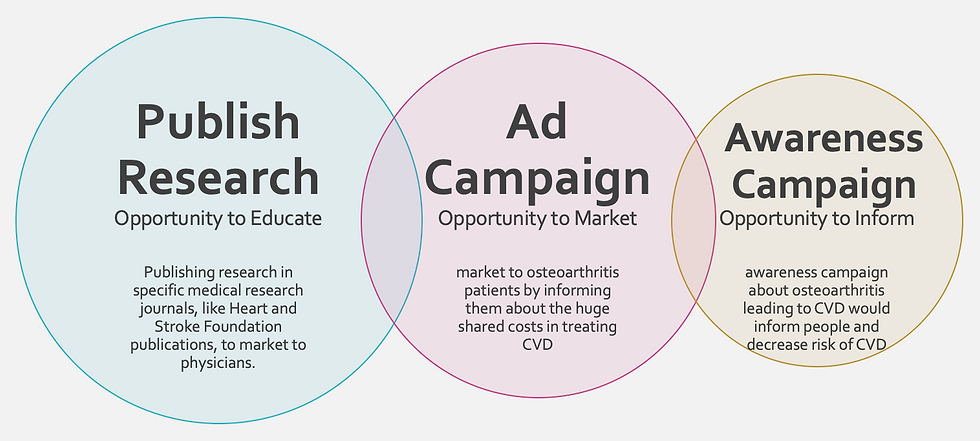
References:
Statistical Society of Canada Case Study 2, organised by Dr. Ehsan Karim, School of Population and Public Health, University of British Columbia (UBC). https://ssc.ca/en/case-study/case-study-2-risk-cardiovascular-disease-among-osteoarthritis-patients
Heart & Stroke Foundation's 2016 Report on the Health of Canadians: The Burden of Heart Failure. https://www.heartandstroke.ca/-/media/pdf-files/canada/2017-heart-month/heartandstroke-reportonhealth-2016.ashx?la=en&hash=91708486C1BC014E24AB4E719B47AEEB8C5EB93E
Comments